Predictive Analytics and Ad Performance
By Heather Fletcher, AdWeek
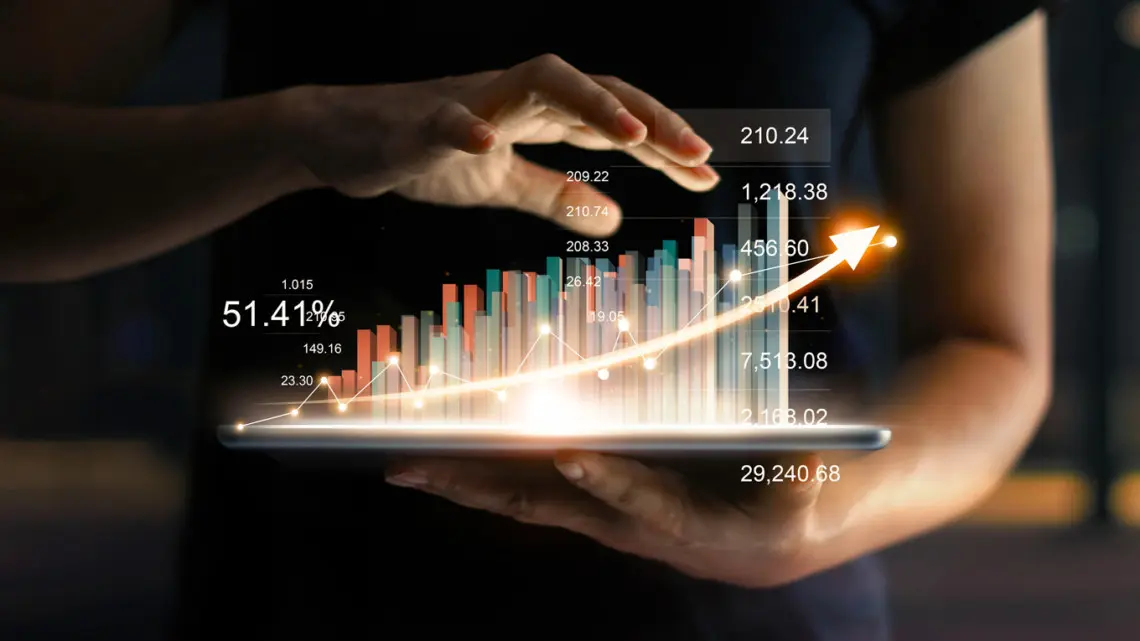
Marketers should first determine why they’re optimizing ads at all.
Everyone loves a sure thing, especially someone who’s paying for an ad. In a world where many business leaders waste money on ads by going with their gut feelings or worse—collecting as much data as possible on ad audiences and inevitably targeting them with irrelevant advertisements—what are the best ways for performance marketers to use predictive analytics to optimize ads?
Performance marketers need to start by figuring out why they’re optimizing their ads at all. Do they want to increase sales? Acquire customers? Accomplish some other goal? This seemingly simple step is where a lot of performance marketers go wrong. They collect all of the data they can instead of the data they should.
Imagine if Karen Heath from Teradata hadn’t wanted to help a retailer increase diaper sales in 1992. She may never have sought out the data showing that when men bought the high-margin item, they also bought beer. By placing beer and diapers together, the retailer’s sales rose. This one finding for one retailer that resulted in product placement changes in 1992 eventually evolved into the more advanced predictive analytics that optimized multichannel marketing in 2011. Now, in 2020, the practice is so advanced the basic definition of predictive analytics says it incorporates machine learning techniques. Predictive analytics practitioner Helen Xiaoqin Yi, a data scientist at a major electronics retailer, suggested performance marketers use “predictive tools to create audience segments or explore new potential audiences” with algorithms like SVM, logistic regression or neural networks. “Then we can analyse their preferences from the comments, reviews, social media, interactions with ads, events or any relevant campaigns, and design several plans for different segments,” Yi said. Stephen H. Yu, president and chief consultant at Willow Data Strategy, advised that performance marketers figure out who should be targeted with which ad and through which channel before personalizing ads. “A series of personas based on propensity modelling can be useful in determining the most optimal offer and creative for each target,” Yu said.
Devyani Sadh, CEO and chief data officer of Data Square, provided three possible segments: 1. Prospects: Identify top-performing prospect ad audience segments based on demographic and psychographic similarities, content preferences, and interests of known high-value customer “clones.” 2. Active customers: Model customers’ prior history along with a “similarity index” of others with similar purchase patterns to optimize ad content. Examples include cross-sell or the next logical product (concurrent or sequential). 3. At-risk or lapsed customers: Stage 1 is identifying those who are staged to attrition or already lapsed, but are likely to respond to an offer. Stage 2 is optimizing ad messaging for retargeting or other initiatives by predicting special offers and promotions most likely to resonate with this group, based on history.
Yi suggested launching a small test of several ad designs using different times of day, durations of exposure and placements in order to prove that the optimization worked. Then, give credit where credit is due. Yu added that performance marketers need to make note of how well each element and channel worked. In other words, don’t default to blanket attribution.
For example, performance marketers will want to keep track of more than just ad placement. Within this one area alone, Sadh said performance marketers can “optimize ad placement by ranking top-performing platforms, affiliates, social media sites, websites, search engines and regions by extrapolating from navigation patterns, search, browsing behavior and digital identities of known converters.
But even a sure thing won’t be a sure thing forever. Just like how search engines regularly update algorithms, performance marketers will need to revisit the predictive analytics process to continue optimizing their ads. Sometimes, marketers will have to start from scratch.
Yi said program or campaign dashboards will tell performance marketers if they need to update the models they build based on the process. “No matter what the results are, we should always summarize and learn from them to prepare for the next campaigns,” she said.
Feature Image Credit: Predictive tools can help brands form audience segments and gain new consumers. iStock